AI value realization has a structured data problem — here's how to solve it
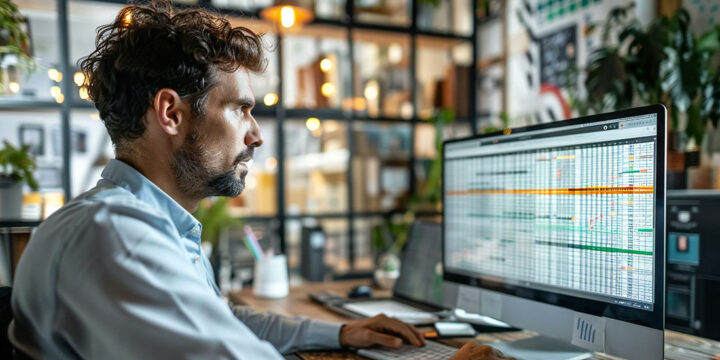
Just a few years ago, the AI first movers owned a competitive advantage. Even poorly orchestrated AI experiences delivered small gains in operational efficiency and self-service capabilities that their competitors simply didn’t have. However, as AI use cases have matured, a new gap has emerged between those organizations that prioritized intentional AI adoption – and those that didn’t.
Organizations that skipped the foundational data integration and governance phases of AI adoption now routinely see their outcomes falling short of their peers.
I’ve talked about this fundamental problem at length before. In short, AI literacy and data literacy are inseparable. To realize the full potential of AI, you need to have sound data practices to back it up. CX leaders agree. According to a recent TTEC Digital report, 73% of CX leaders now say data quality has a moderate or major impact on their ability to achieve their goals using AI.
So, the question becomes: What does quality data look like and how do you build a data strategy that feeds better AI outcomes?
For the past couple of years, the conversation has shifted to unstructured data strategies. That’s because this was the data necessary to get value from emerging AI use cases, often centered around basic text generation, summarization, search, and Q&A.
Today, unstructured data can be activated for AI use cases through a proven blueprint called Retrieval Augmented Generation (RAG). RAG takes your documents, creates embeddings from them, stores those embeddings in a vector database, and queries them. Companies then optimize RAG solutions for speed, accuracy, and price.
Looking for support with your unstructured data? We can help.
Contact one of our data experts to see how we can put your unstructured data to work for you.
And yet – even after organizations have executed this complex process for their unstructured data – they tend to have trouble providing the necessary context for their AI models to actually generate meaningful insights from it. Why?
Often, it’s because they haven’t considered how their structured data fits into the generative AI picture. Instead, there is an assumption that because this data is already “structured,” it is prepared to support AI use cases.
Why structured data is a critical problem to solve for large language models (LLMs)
While structured data only makes up about 20% of the average organization’s data pool, there are two reasons why it is a mistake to overlook revisiting your governance of it.
#1: Chesterton’s Fence.
If you’re unfamiliar, Chesterton’s Fence is a philosophical principle that stems from a parable from GK Chesterton’s 1929 book, The Thing. It asserts if a fence has been erected somewhere, it was likely done for a reason. For example, maybe there was a dog on the other side the builder hoped to pen in?
Think of structured data the same way. If it was deemed important enough to painstakingly compile into spreadsheets once, it’s likely because it’s critical business data you would need to solve many important business challenges.
Using data to solve business problems is both art and science. For complex business problems, we need to move between both structured and unstructured data to make decisions. When we feed a large language model unstructured data alone, we risk limiting its decision-making abilities, falling short of our targeted productivity goals.
#2: There’s a false assumption that structured data is already ready for LLMs.
To illustrate this point, let’s create a hypothetical example. Say you’re a healthcare provider, and you’re focused on improving revenue cycle management (RCM) by increasing the likelihood of accurate timely billing and reimbursement.
Using your structured billing dataset, you ask your LLM which insurance provider has the longest average payment time for orthopedic claims. This should be a simple RCM question, especially since you already have all the data laid out in beautiful columns.
But the structured data lacks some critical content that would allow the LLM to correctly use it. For instance, the data is organized by bill codes, but those codes are not defined anywhere in the spreadsheet. As a result, the AI model can’t answer the question as it was asked because it doesn’t know which claims are orthopedic. Additionally, the time payment column doesn’t specify if it is tracking in hours, days, or months.
When you extrapolate this example across the two, three, or ten datasets that might be used to answer a single question, the sheer number of potential semantic issues can create big problems for AI performance.
Empowering structured data to drive AI value realization
This brings us to how we actually solve this problem. All of these disparate data sets, both structured and unstructured, need a common framework for data analysis. Creating a semantic layer for the data is the answer.
A semantic layer sits between the technical data (spreadsheets and tables) and the end users who need to use it. It provides a consistent and coherent view of the data across the organization – breaking data silos and standardizing the data so that it can be applied to business problems more effectively.
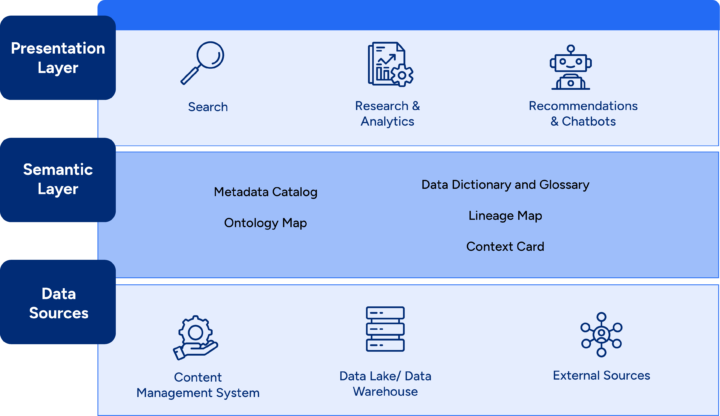
When it comes to building a semantic layer to sit between your unstructured data and your use cases, there are generally three key components to consider.
- Business context: The heart of the semantic layer. Business context transforms structured data into something meaningful for generative AI models to use. By making sure the full relevant context of your structured data is known, documented, and even annotated alongside the dataset, you can establish a clear model for using the data. To create this context you may need to build ontologies, knowledge graphs, and other metadata catalogs.
- Data governance: It’s important that the data doesn’t drift too far from its original purpose over time. Governance guardrails will help ensure availability, integrity, and security of the data assets used by generative AI models.
- Prompts and embeddings: Depending on the scale and variety of data used, a solution might rely on prompts or other techniques to inform the generative AI model about the relevant characteristics and details. By curating these details – with business context and data governance – into a format that can be accessed by the models and updated by humans as needed, you provide your AI model an instruction manual for how to engage with your data in the semantic layer.
Nearly 90% of AI pilots fail to make it to production, according to IDC research. That’s not a typo – AI pilots are much more likely to fail than to succeed.
But which pilots fail, and which pilots succeed, isn’t random. It’s all right there in the data.
Without strategies for both unstructured and structured data, organizations risk creating AI-enabled experiences that fall into the first category. For those that get it right, however, AI can become a powerful differentiator that drives operational efficiency, incremental revenue, and long-lasting customer loyalty.
We can put the 'structure' in your structured data
At TTEC Digital, we have the right combination of experts to design a powerful semantic layer strategy for your business. Let’s connect to see how we can help your organization get more strategic about structured data.
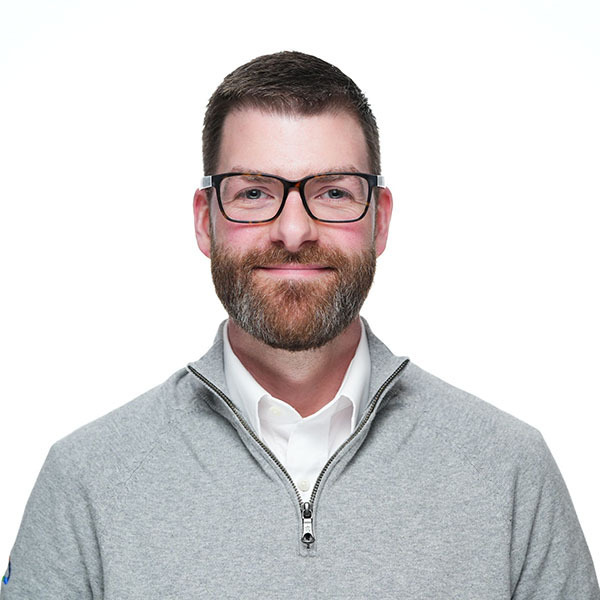
About the Author
Aaron Schroeder
Director, AI SolutionsAaron aids clients in leveraging AI to enhance speed, consistency, and innovation in customer experience.
Read moreJoin our mailing list
Receive exclusive updates on the latest CX trends, events, and solutions.